
Machine Learning (ML) for Uncertainty Quantification (UQ)
Forward UQ

Inverse UQ


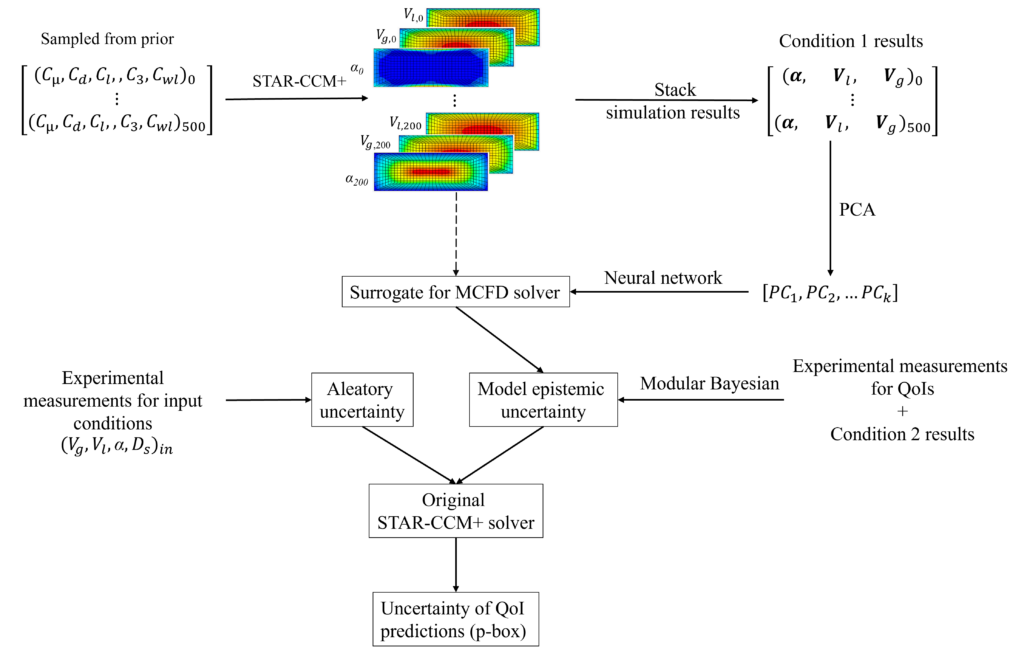
UQ for ML
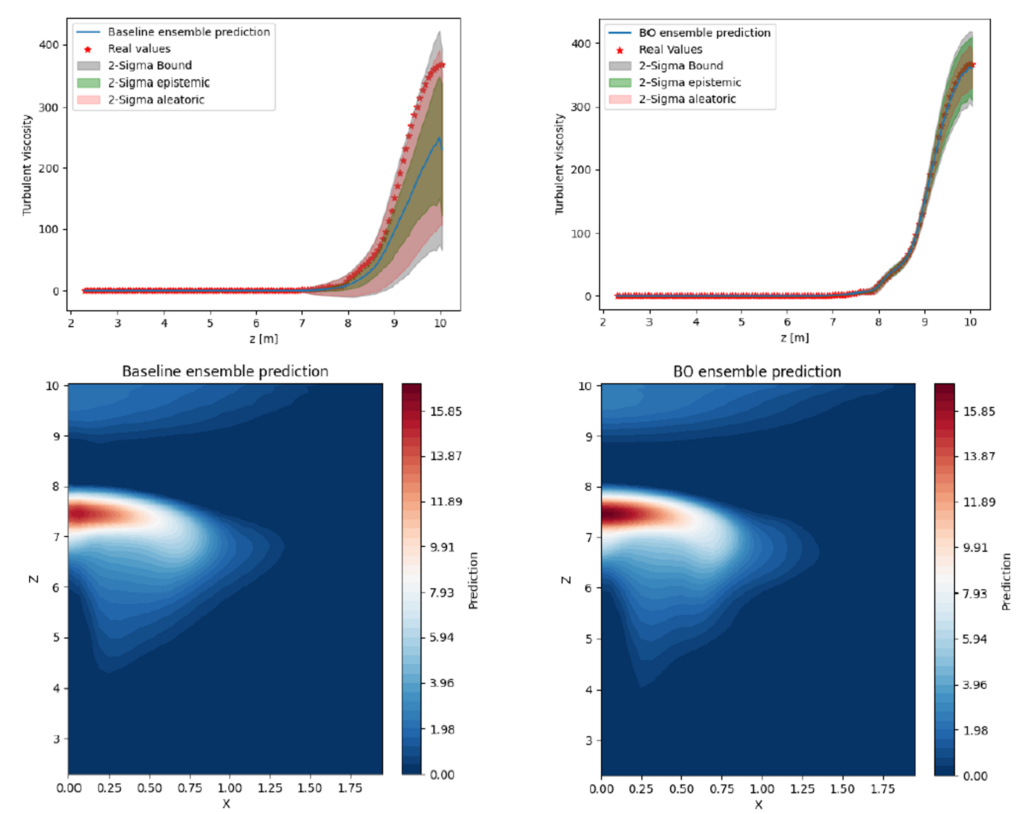
Selected Publications
- Liu, Y., Dinh, N., Smith, R.C., & Sun, X. (2019). Uncertainty quantification of two-phase flow and boiling heat transfer simulations through a data-driven modular Bayesian approach. International Journal of Heat and Mass Transfer, 138, 1096-1116. DOI: 10.1016/j.ijheatmasstransfer.2019.04.071ke
- Liu, Y., Sun, X., & Dinh, N. (2019). Validation and uncertainty quantification of multiphase-CFD solvers: A data-driven Bayesian framework supported by high-resolution experiments. Nuclear Engineering and Design, 354, 110200. DOI: 10.1016/j.nucengdes.2019.110200
- Liu, Y. & Dinh, N. (2019). Validation and uncertainty quantification for wall boiling closure relations in multiphase-CFD solver. Nuclear Science and Engineering, 193, 81-99. DOI: 10.1080/00295639.2018.1512790
- Wu, X., Liu, Y., Kearfott, K., & Sun, X. (2020). Evaluation of public dose from FHR tritium release with consideration of meteorological uncertainties. Science of the Total Environment, 709, 136085. DOI: 10.1016/j.scitotenv.2019.136085
- Liu, Y., Wang, D., Sun, X., Liu, Y., Dinh, N., & Hu, R. (2021). Uncertainty quantification for multiphase-CFD simulations of bubbly flows: a machine learning-based Bayesian approach supported by high-resolution experiments. Reliability Engineering and System Safety, 212, 107636. DOI: 10.1016/j.ress.2021.107636
- Liu, Y., Mui, T., Xie, Z., & Hu, R. (2023). Benchmarking FFTF LOFWOS Test# 13 using SAM code: Baseline model development and uncertainty quantification. Annals of Nuclear Energy, 192, 110010. DOI: 10.1016/j.anucene.2023.110010